The phrase, “quantum machine learning” is gaining public traction – here’s why.
Machine learning (ML) has undoubtedly captured the public eye. A simple search for “AI” returns well over 25.2 billion results. The area has been called “revolutionary” for fields spanning from computer science to elementary education. So how does AI fit in quantum computing?
The excitement around AI is still growing: it has intrigued people at every level of society, and its disruptive potential has generated excitement. This feeling – of breaking from the norm – has leaked over into the world of quantum computing research. Following a jump in interest in quantum computing over two decades prior – which itself followed Shor’s proof of a prime factorization algorithm capable of being more efficient than a standard computer – a series of proposals suggested the idea of a “quantum neural network” (QNN) in as early as 1995, if not before. This is occasionally referred to by the umbrella term quantum machine learning (QML).
What is Quantum Machine Learning?
Quantum Machine Learning (QML) is the intersection of quantum theory with that of machine learning – and more specifically, often neural networks. In early implementations of quantum machine learning models, researchers struggled against working with nonlinear problems, as the low level of qubits in theory meant most problems were constrained to linear solvers.
What’s a linear problem? These are mathematical representations of a real-world (or simplified real-world) problem that can be written in the form Ax = b, much like the ax = b line on the Cartesian-coordinate plane that may be encountered in a middle school math class, except in more dimensions than just one or two.
The idea, then, was to figure out a way to represent the distribution of the training data that used quantum properties. The unique method of information representation in quantum computers relies on the phase of the qubits – exactly what this means depends on the type of quantum computer, but generally, this refers to the value of a quantum property known as spin.
Early QNN models could, for example, learn the data distribution of some input and use the possibility of measuring some series of outcomes to correspond to the given data distribution. This learning process would make use of a generalized version of the famous Grover’s search algorithm.
Read also: Grover’s Algorithm Using 2 Qubits: Step-by-Step Guide
Why the Spotlight on Quantum Machine Learning?
To fully understand the excitement around quantum, we start our discussion with the struggles of QML and quantum computers in general – and why investment in QML is worth it in the long run.
Acknowledging the Struggles of QML
The use of iterative, variational algorithms means QNN’s are incredible for learning smooth, repetitive patterns – that is, data that has “harmonic” features (think: harmonic, like repeating sinusoidal functions). However, some QNN implementations struggle terribly with data that tends to not be harmonic. This is almost the opposite of the struggles with typical computers. Neural networks on these classical computers tend to struggle with harmonic features in the training data, instead being quite incredible with nonharmonic features, which contain less of a smooth, repeating pattern.
To remedy this, researchers have leaned into hybrid algorithms – such as parallel hybrid networks – that capitalize on the strengths of both quantum and classical computers. These have been shown to have advantages over variational QML algorithms and their classical equivalents operating on their own.
The final, overarching struggle against QML lies in hardware. As with any quantum computer, hardware limits the implementation of theory of any quantum algorithm. Simple gates – such as a multi-controlled NOT gate – can equate several dozen low-level, simple 1- or 2-qubit gates. However, many quantum computers lack sufficient qubits to implement this. Furthermore, they struggle to sustain an environment without the inevitable loss of significant information in the bits (incoherence).
Error-prone quantum computers have made hybrid algorithms of particular interest, but the hope is to develop hardware capable of running increasingly complex algorithms or to compute results for larger inputs with time.
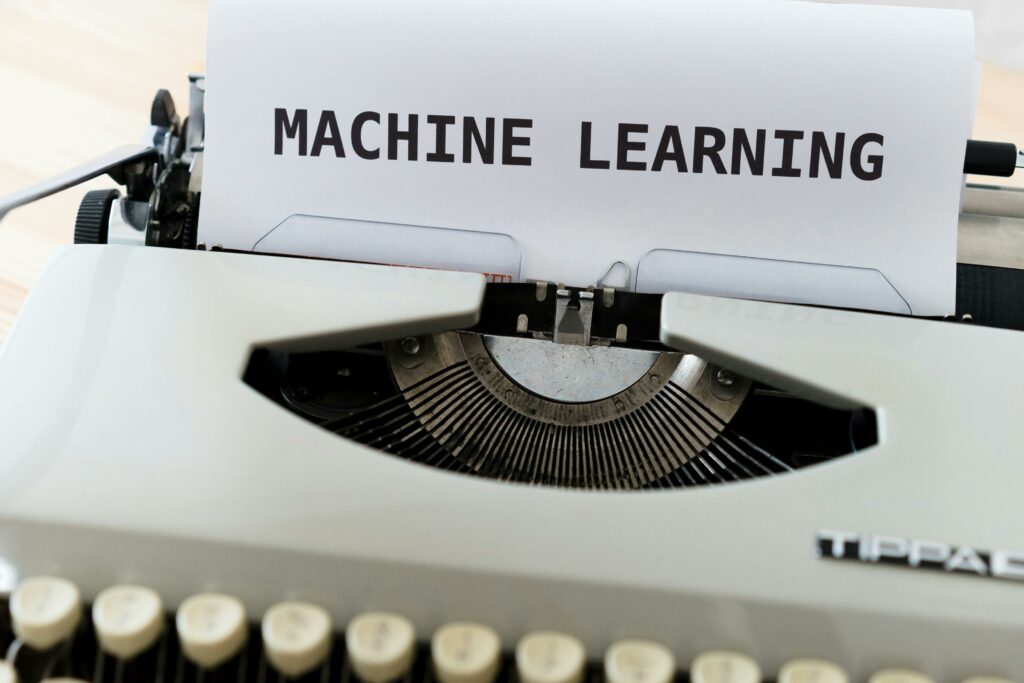
So… Why Are We Excited About Quantum Computing Machine Learning?
Researchers are working around the clock to find error correction and mitigation techniques – on top of hardware architectures – that can help reduce some of these hardware-based concerns.
The excitement around quantum machine learning lies in the ability to consider a significant number of phases (states) simultaneously. As a result, rather than having to rely on repetitive training epochs, QML algorithms could hypothetically resolve the problem in a single “turn.” This is exemplified through efficient quantum least squares fitting – one of the most common optimization problems encountered on the regular. This simplified framework also translates to higher energy efficiency.
For machine learning algorithms that make use of Shor’s algorithm or Grover’s algorithm, they obtain the proven advantage over classical computers from the algorithms they are respectively based on. Admittedly, these may only be applied to a specific subset of problems that may be phrased in a way that is a valid input to the respective algorithms. The prospect is nonetheless exciting, and acts as steady evidence for the potential advantage quantum computers provide as a whole.
Yet the advantages extend beyond reduced complexity or increased efficiency. In March 2024, researchers at the Freie Universität in Berlin, Germany showed astonishing results: in some settings, QML algorithms were good at learning seemingly random data. The significance of these findings can not be understated – learning the idea of “random” data that lacks patterns is a clear struggle of many ML algorithms, as these rely on training methods that gradually evolve to a certain shape over time. Existing QML models were capable of labeling seemingly random input states, the equivalent of almost memorizing the data.
This follows from earlier results referring to studies that revealed QML algorithms could be trained on “surprisingly simple data” – suggesting near-term applications for quantum computers could be more within reach than believed.
As such, the possibilities are extensive – warranting increasing attention as AI and quantum hardware continue to grow.
What’s Next for Quantum Machine Learning?
All in all, quantum techniques will continue to mature. As such, researchers are putting more attention into developing robust algorithms that step beyond the typical notions of machine learning. They continue to find trends that emerge in using quantum computers for ML model construction. This continues to motivate the driving question of any quantum computing research: what happens when computations aren’t limited to 1’s and 0’s anymore?
Disruptive work, indeed.
Additional Resources
For a more in-depth explanation of classical and quantum machine learning, check out Quantum Insider’s technical explanations, broken down, or explore the Q-munity Tech website at LINK.